Trust in Autonomous Vehicule and AR visualization
Improving autonomous vehicle drivers’ confidence via Augmented Reality visualization of artificial intelligence decision-making context
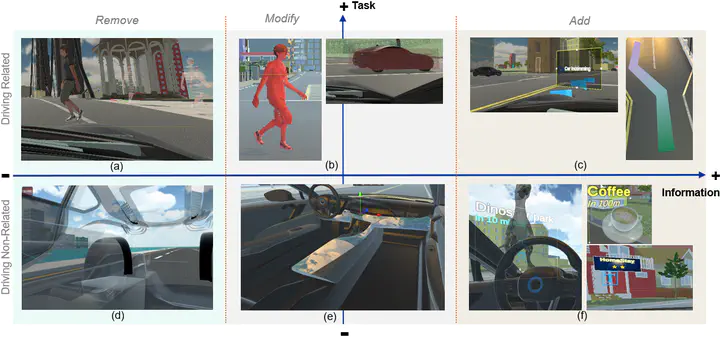
Abstract
This PhD project aims to enhance trust in autonomous vehicles by improving driver situational awareness through augmented reality (AR). Recognizing that unpredictable AI behavior and sudden transitions from autonomous to manual control can cause discomfort and safety concerns, the research will develop AR techniques to visually communicate the vehicle’s decisions and potential hazards to the driver. This approach will help drivers understand and trust the vehicle’s actions, especially in critical moments when manual intervention is needed. The project will tackle challenges in real-time hazard visualization and decision transparency, ultimately aiding the deployment of level 4 and 5 autonomous cars.